AI and you:
where to begin?
How to successfully implement
AI in businesses - Part 2
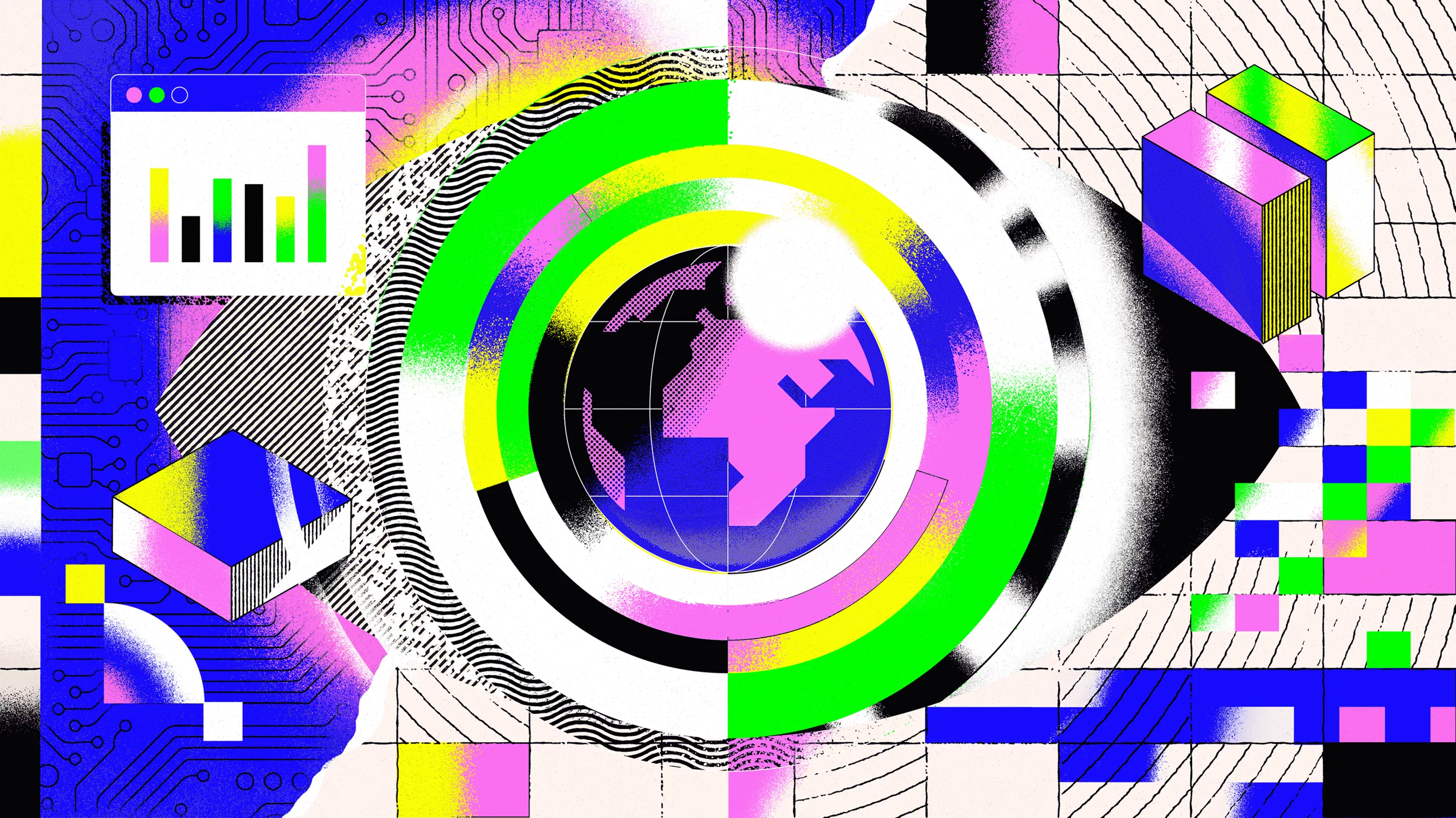
Increase efficiency, speed up processes, and relieve employees from manual work: most managers have by now recognised the fundamental relevance of artificial intelligence. So far, so good. But how do we take the next step? Or rather, the first step: namely, the actual introduction of AI in one's own business?
Here, many managers are faced with a riddle. What exactly is AI capable of? How could it affect my product, my business model, and my industry? And most importantly, where specifically can I use it profitably in my company?
In this post, I will introduce you to different approaches in order to find out exactly that.
Where do you begin?
There is one important factor that you should not forget amongst the media hype about artificial intelligence: AI is not an end in itself. Artificial intelligence - data science, machine learning, whatever you want to call it - is a tool in your entrepreneurial toolbox. Used sensibly, it is extremely effective. In the midterm, it will become indispensable. But there is no universally applicable, one-size-fits all solution.
Where and how AI can be used most effectively varies from company to company. So if artificial intelligence is not to be used just for the sake of using it, it must instead be applied in a meaningful, beneficial and value-creating way. The individual characteristics and USPs of your company must be taken into account. To do this, you must weigh up the following points:
1. Strategic Objectives
1. Strategic
Objectives
Anyone who wants to use AI should be very clear about the goals he or she is pursuing with it. You probably want to save costs or increase your sales performance. Perhaps you want to standardise and accelerate your processes. Or maybe you would like to mitigate the current skilled labour shortage and the impending loss of experience-based knowledge. In any case, you should make sure that your deployment of AI is a targeted continuation of your business strategy.
I recently chatted with a provider of an AI-based logistics optimisation software. The company's tool is in high demand: numerous companies want to optimise their delivery routes with the help of artificial intelligence. But the incoming requests all sound similar: please use the technology to make what already exists "better". Unfortunately, there is not much that the software provider can do with that. What exactly does "better" mean?
For example, optimising the route of a logistics truck can mean keeping transport costs low or saving CO2. On the other hand, the route could also be planned in such a way that the drivers have as pleasant a trip as possible, thereby preserving their working capacity in the long term. Having a clear strategic objective is a prerequisite for the effective use of AI. "Better" is not a strategy.
2. Determine the potential for
innovation
Once you have defined your strategic goal, start by taking a closer look at individual components of your business. Where would targeted innovation help you achieve your strategic goals? And what could AI do to help? This is how you can identify areas with the potential:
Within the product
The use of AI makes sense here if ...
…it can be used to improve existing products or develop new ones, thereby fulfilling the needs of the target group more comprehensively, or opening up new markets. For example, if product functionalities can be personalised.
At first, this may sound dry. So let's look at an example: Let's assume you are running an energy company. Of course, your product is so much more than just the electricity that you offer. To stand out from the competition, you offer not only the energy your customers need, but also personalised, usage-based insights. Using data and AI, you’ve developed a digital platform that helps private households improve their energy efficiency. Here's how it works: electricity consumption is measured via a smart metre. AI analyses the electricity data, recognises all the consumer devices in the household, and provides customers with tips on how to save energy. This is done via the platform, and is broken down per device in the household. Thanks to AI, you can live more energy-efficiently. Now that’s real added value.
Alongside your core processes
The use of AI makes sense here if ...
….internal production processes can be optimised through the use of data and artificial intelligence, without changing the product or service itself. The products and services are created according to the original strategic goals - but they now work more efficiently, are more scalable and/or more resource-efficient.
What could that mean for your energy company? Via digital technologies, including smart metres, you can record your customers' energy consumption in real time. Based on this live data (and other specific information about the household and the building), the AI creates forecasts for individual consumption patterns. Additional data such as changes in weather, energy prices and mobility information also flows into the forecast models, enabling even more accurate predictions.
Now let’s think another step further: with the help of AI clustering, you can identify groups that have similar usage behaviours. This enables accurate forecasts for large customer groups and ultimately for the entire power grid. The result: from now on, you can adjust energy production to the forecasted demand for electricity - thereby maximising efficiency and security of supply. Both bottlenecks and surpluses are avoided. This becomes an active and important contribution to achieving the energy transition.
Alongside your support processes
The use of AI makes sense here if ...
…support processes (customer support, finance, human resource management and infrastructure management) can be optimised through the use of AI and data analytics. This results in cost savings, improved performance and a reduction in errors. In short: smoother and more efficient operations.
Of course, this also applies to the support processes of your business. The key word here is security of supply. Both the physical infrastructure (power grid) and the digital (cyber security) are optimised, expanded efficiently, and protected by AI. AI also helps with predictive maintenance - the prediction of maintenance windows. Drones and image recognition AI can also be used to maintain infrastructure that is difficult to access. This results in a clear gain in efficiency and improvements in the security of personnel and supply.
New business models
The use of AI makes sense here if you are willing to leave behind the avenues of thought and action that you are familiar with. After all:
You are developing innovative business models in which data and AI no longer only have a supporting function, they become the core of the product or service. These approaches open up completely new ways of monetisation and enable the development of previously unserved or newly emerging market segments.
Imagine that you have successfully implemented AI in your company following one of the above approaches. From now on, you collect data and insights that other companies can only dream of. So why not make a business out of it? You develop a SaaS B2B product called "Smart Business", based on the data you have collected and the existing AI models that are in use within your business. For example, your software now helps other businesses adapt their production phases to the forecasted electricity prices. AI also uses data to benchmark your performance to other players in the market, and provides tips on how to optimise your business beyond just energy consumption.
The exciting part is: You not only sell this new software to your existing clients, you even licence it out to the competition. So while your competitors are trying to keep up with you, in the meantime, you are profiting from this.
And that’s not all: Because your business is helping other businesses optimise their processes using AI, "Smart Business" is helping reduce the overall CO2 footprint and thereby promoting an overall more cost-effective and resource-efficient energy usage.
3. Reality check: From theories to facts
That’s all fine and good, you might say. Four approaches, four effective examples of how this might be implemented. But how do you develop an idea of how to implement AI in your business, where things might be looking very different? That’s a very good question.
The practical application of these four approaches
In innovation processes, the usual procedure looks like this:
First, you choose one of the above areas. Then, within the boundaries of the respective area, you develop ideas on how and where AI could be used.
Only later, in a second step, you start considering aspects like technical feasibility. Then, in a worst case scenario, IT and data experts are called in, who then explain to you that your creative ideas unfortunately have nothing to do with the current capabilities of artificial intelligence ... Or that there is nowhere near enough existing data to build an appropriate model.
Or the other way around: again and again, I observe how specific departments (especially within support departments) demand that well-known applications such as ChatGPT are used to usher in quick improvements of existing processes. Naturally, we seek what we are familiar with – even if there are better options available. Tragically, but logically, this leads to another realisation: we cannot seek something that we don’t know exists.
But this can come at a cost: Without the right knowledge of technical processes, you run the risk of purchasing AI models without having an awareness of potential follow-up costs. Personnel, infrastructure, data, and resources: all these are costs that should be considered in advance. Otherwise the AI rush will quickly end with a painful hangover. The bottom line is - this approach is both strategically and economically unproductive.
No matter how you try to twist and turn it - this is an absolutely decisive moment in idea generation: Not every wish is technically feasible. And not every option that you know of, or that is technically feasible, makes economic sense.
4. A combination of relevant factors
In my view, introducing AI in a company will only succeed if, in addition to the previous steps, further considerations are integrated into the process: especially, factors beyond one's own limited perspective or area of responsibility. You even need to consider factors that go beyond your own company, and beyond your own area of expertise.
Which factors are these? To roughly categorise them, they are: economic viability, technical feasibility, data availability and customer needs. These questions should never be ignored at any time during the decision-making process. What is the relevance of your product for your customers? Which processes are profitable, which are not? What is technically feasible? And is all the necessary data available?
As soon as I start looking for potential areas for innovation with my clients, I compile questionnaires on each of these factors.
Only when you look at an idea from all relevant aspects will you be able to recognise its strengths and weaknesses. Most AI consultancies focus on one, at most on two of these factors. All others remain blind spots.
I am convinced that many consultancies therefore fall short. In order to find sensible, beneficial and economically viable applications of artificial intelligence for your company, all of the above-mentioned points must be considered equally, simultaneously and holistically.
When evaluating possible AI use cases, it is not just about finding the one right approach. It is always about combining all the different factors in a profitable way. It goes without saying that this cannot be done alone.
5. Finally: Get started
Aside from economic efficiency, at PLAN D, we therefore include the factors of "data" and "technology" in our innovation processes from the very beginning. We do this not only in order to explore technical feasibilities and economic appropriateness at an early stage. We include these factors so that we can come up with other ideas in advance, in order to broaden our mental horizons and expand the playing field for creativity.
Did you know that due to the upcoming Data Act, incredible amounts of data will soon be on the market? Are you aware of the extent to which AI can process data? Or what types of functionalities could be attainable with the corresponding data? Have you ever thought about the direction in which your current business model might develop as a result of combining data with technologies you don't even know exist yet? No? Well, how could you?
In order to do this, you need information. And the way to get this information is by bringing the right people together at the same table. Data scientists and industry experts, IT professionals and project managers. I myself have made it my job and mission to search for the best and most beneficial possible applications of AI in companies. For this purpose, I have assembled a diverse team of the very best minds from a huge variety of disciplines. And I can promise you one thing: Never has my profession been more exciting, and never have the entrepreneurial possibilities been greater. Honestly, getting started has never been more fun than it is right now.
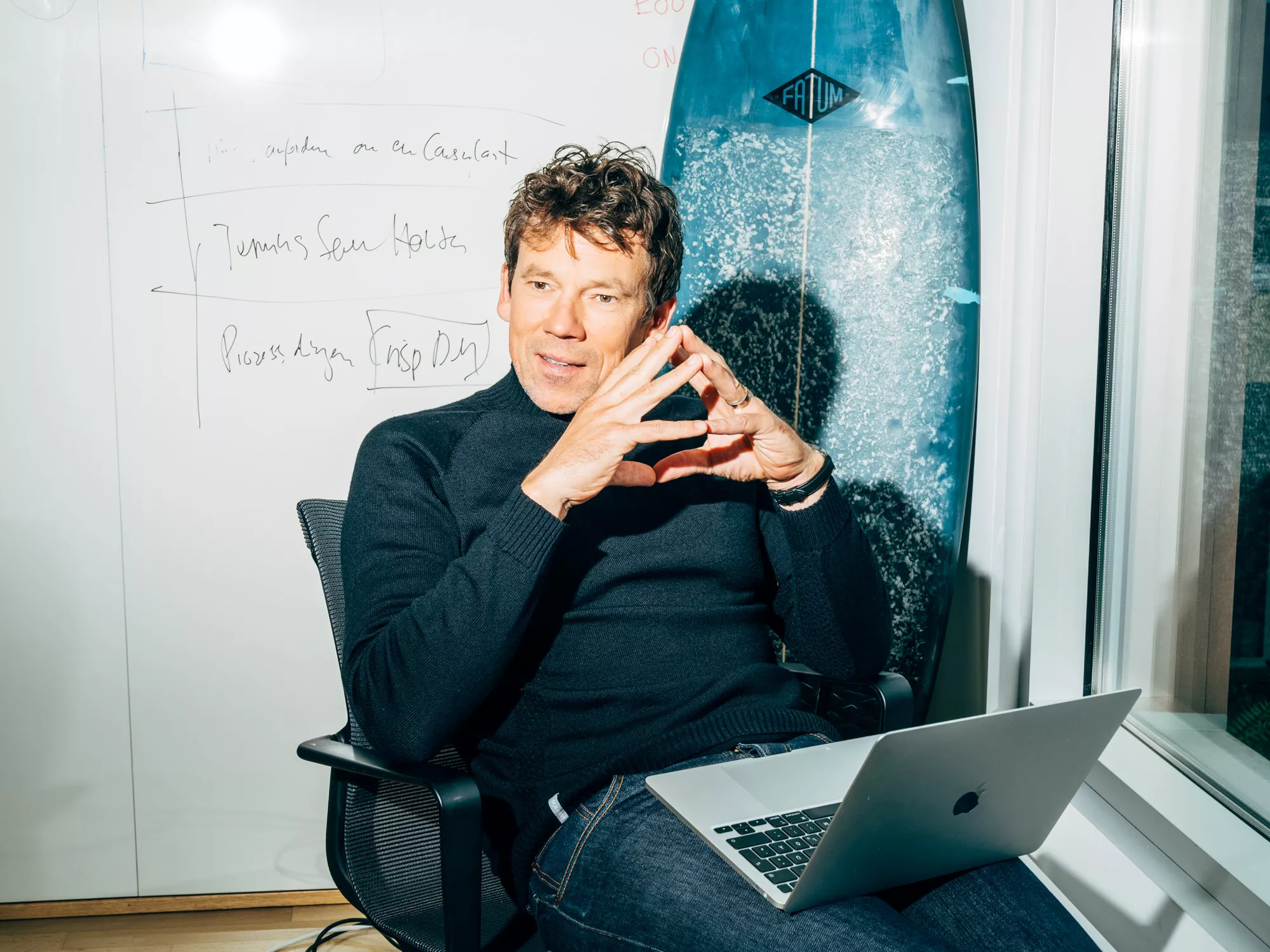
Further information
If you now want to get started, too, I have a small piece of advice for you here. Try to become aware of the entire scope of strategic possibilities available to you. I always offer my clients a two-day crash course in AI and its possibilities, so that they can build up a sound basis for decision-making.
This is not only about exploring the existing state of technology, but it also entails a highly focused deep-dive into the perspectives and goals of our clients: What is the value of data specifically for our client’s industry? What are the options for data collection? And how might this impact their particular business model?